Biggest Problems in AI Today How to Solve It?
“Artificial intelligence is the new electricity.”
This statement by Andrew Ng, co-founder of Google Brain and Coursera, is indeed true. The global AI market is also on the rise and is expected to add $15.7 trillion to the global economy by 2030, as stated in a PwC report. However, issues such as data bias and regulatory challenges are still hindering full adoption.
The emergence of Artificial Intelligence has changed the face of many industries. The automation capabilities have revolutionized many industry processes. With the collaboration of other techniques, we are heading toward Industry 4.0, commonly known as the Fourth Industrial Revolution.
However, AI is still in its infancy stage, and as we progress towards its evolution, a list of problems is emerging. We have a long way to go to understand the true potential of artificial intelligence. For instance, let’s consider the issue of bias. This issue can happen if the training dataset is improper, and it can lead to the generation of unfair and inaccurate results.
This is just one example of the challenges in AI, and many such issues need an apt solution to overcome the hurdles and make AI more efficient and better. However, these challenges stand as the stepping stones to making significant progress on the way to understanding AI’s true potential.
Challenges in Artificial Intelligence
There are many problems or challenges in the artificial intelligence field that need to be solved from a new perspective using a different approach. Let’s see some of the challenges:
Lack of Explainability
As we progress towards AI adoption, there will be AI systems that will make key decisions. For instance, an AI system that can diagnose a condition based on the symptoms and patient’s medical history, or an AI model deciding whether a candidate should be given a loan or not based on the credit score and other factors.
However, the challenge is how a professional will come to know whether AI has made the right decision. How will an AI system be able to explain on what basis it has predicted or provided the given decision?
By 2030, AI will help make clinical decisions in more than 40% of cases; therefore, transparency is even more important now. To overcome this problem, explainable AI (XAI) models are being designed and developed to provide clear and concise explanations for their decisions and predictions.
2. Catastrophic Forgetting
This is another common issue researchers have found while trying to build AI models that focus on continuous learning. Catastrophic forgetting is a phenomenon where the AI model forgets the previously learned information after acquiring new information.
Researchers have devised various techniques to overcome this problem. These techniques mainly include progressive neural networks, gradient episodic memory, and lifelong learning forest models.
3. Difficulty in Replicating Lab Results in Real-Life
In 2016, Researchers from Google’s AI division DeepMind and the University of Oxford developed the most accurate lip-reading software. After thousands of hours of training on TV footage from the BBC, scientists trained a neural network to annotate video footage with 46.8% accuracy, compared to 12.8% accuracy for a human professional when tested on the same footage.
Similarly, an AI-based breast cancer scanning system built by Google Health and Imperial College London has fewer false-positive results than two certified radiologists.
No doubt, when AI algorithms are trained using high-quality training data and powerful hardware, they can perform a wide range of tasks with precision and accuracy that even outperforms humans. However, these results are only limited within the laboratory walls. The organizations are inefficient in replicating their results outside the controlled environment.
In 2024, Stanford University researchers found that only 30% of the AI outcomes that are generated in lab environments are applicable in the real world. The solution to this problem lies in the hands of organizations that share the complete research and source code with AI developers and scientists, who interpret it and contribute their efforts in making it work outside the controlled environment.
4. High Computing Power
Another significant AI challenge is the computing power an AI model requires to perform efficiently. According to Professor Mark Parsons, Director of EPCC, the supercomputing center at the University of Edinburgh,
“Hardware is already a bottleneck for AI. After you have trained a subset of data on one of the GPUs, you have to bring the data back, share it out, and do another training session on all GPUs, which takes huge amounts of network bandwidth and works off GPUs.”
Let’s consider ChatGPT. Every query run through ChatGPT utilizes a hefty serving of computational resources. Estimates suggest that it consumes around 3Wh of energy per query, which translates to roughly 0.0018 kWh. Now, this might seem not very important, but it is essential to keep in mind that this value resonates with only one query. Given the daily interactions with ChatGPT, the collective costs become colossal. A report estimates that it takes $700,000 daily to keep ChatGPT up and running.
As the LLM becomes more complex, resource consumption will rise. The only solution to this AI challenge is the adoption of sustainable practices. Overall, the problems will come as we move ahead on the path of evolution, but innovative ideas are required to solve the issues on a large scale. This is where the role of startups becomes critical.
How Startups are Solving AI-Based Problems?
Every problem comes with a solution. A 2024 report by the Data Security Council of India (DSCI) also reveals that 70% of Indian startups are focused on tackling AI-related challenges. These challenges primarily include privacy, irrelevancy, transparency, unethical approaches, and bias.
Startups understand that the power is not in the technology but in how AI is used to handle different problems. Hence, the role of research and development becomes pivotal, which focuses on contextual applications of AI in solving specific problems, like LLM and neural networks.
For instance, Stardog has developed Voicebox to solve the explainability issues associated with AI-driven decisions. This AI-powered data assistant provides clear and explainable answers quickly whenever a question related to the company’s data is asked. This makes it simple for employees to fetch any information related to the company’s operations, like what were the top four products in the last quarter? and similar questions.
To combat the energy efficiency issue, Mistral AI, a French AI startup, has launched Ministral 3B 24.10. It is ideal for low-power, low-latency on-device computing and edge use cases. They also have Mistral 7B model, which is engineered for superior performance and efficiency. This model uses grouped query attention (GQA) for faster interference combined with sliding window attention (SWA). This helps in handling large sequences of arbitrary length with a reduced interference cost.
Emerging startups with innovative approaches to solving problems in the AI/ML field need to be precise about their target audience, customers’ specific pain points, and how their solution will fit into the existing ecosystem. Startups evolving in the field of AI are understanding the problem space and coming up with precise solutions that aim to resolve the problem.
For example, AI can be used to translate one language into another. An Indian startup called VisualDub utilizes this, and it translates an entire advertisement into another language without any reshoots. Their Gen AI proprietary model transforms the face under the eyes, including jaws, mouth, chin, smile lines, and micro muscles in the cheeks and upper neck, to sync the recorded voiceovers with lip movement and visual cues.
Startups have the idea; however, scaling might not be easy for them, but accelerators and incubators like MATH T-Hub can help. MATH T-Hub is the world’s largest startup ecosystem. Their programs, like AI Scale-up program, provide dynamic space, physical resources, like a mini data center with GPU capabilities, and financial support to AI Startups so that they can thrive and scale their services.
The Bottom Line,
These days, bias in algorithms, scalability constraints, and data privacy are some of the significant issues facing artificial intelligence (AI). These problems hinder its wider acceptance and effectiveness. For example, a report by the National Institute of Standards and Technology (NIST) highlights that AI systems can inherit and amplify biases present in their training data. This eventually leads to skewed representations or unfair treatment of diverse demographics
Companies that partner with T-Hub, a preeminent innovation ecosystem, can benefit from its know-how in promoting tech solutions. Through creativity, moral guidelines, and expandable infrastructure, T-Hub’s varied network of entrepreneurs, financiers, and business professionals offers help by all means necessary to help your startup.
By working together, startups can solve the sector’s pressing problems by accelerating AI development, assuring ethical usage, fostering growth, and upholding confidence across industries.
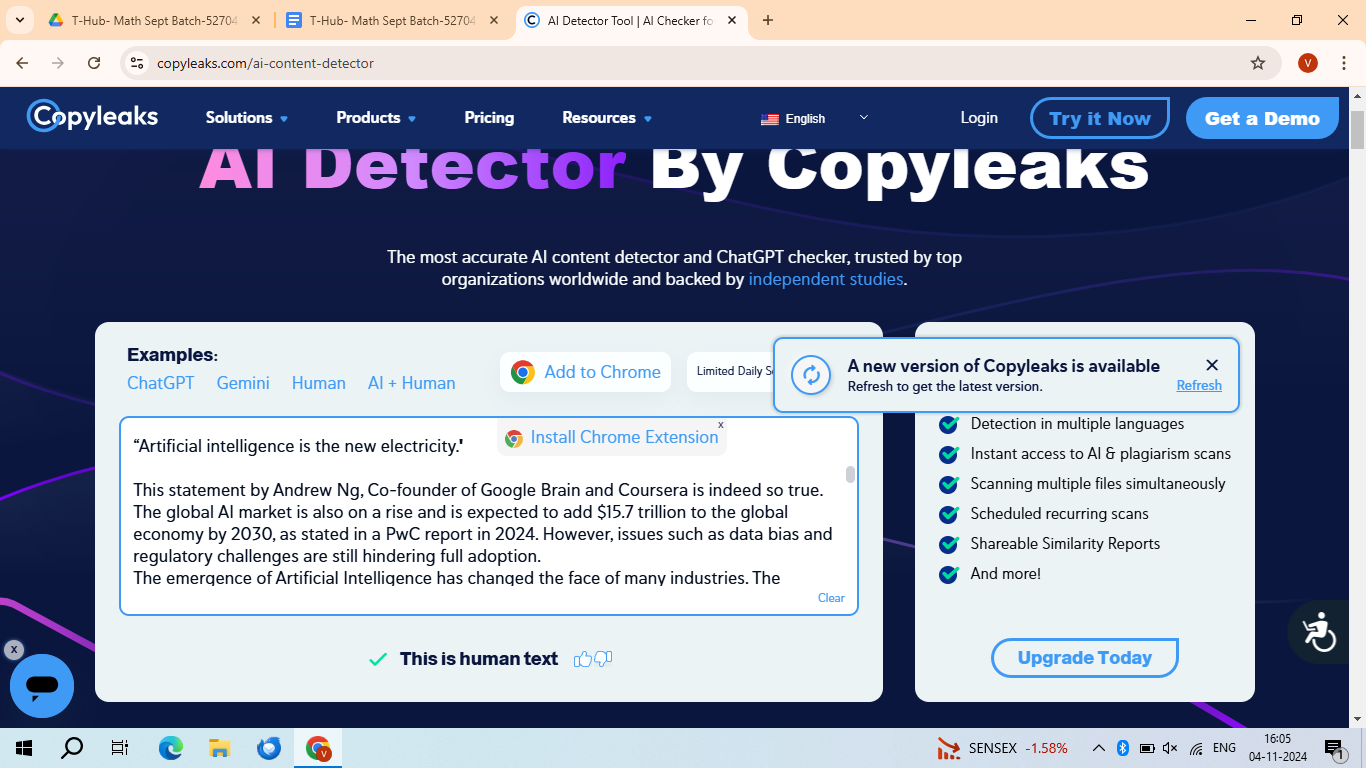
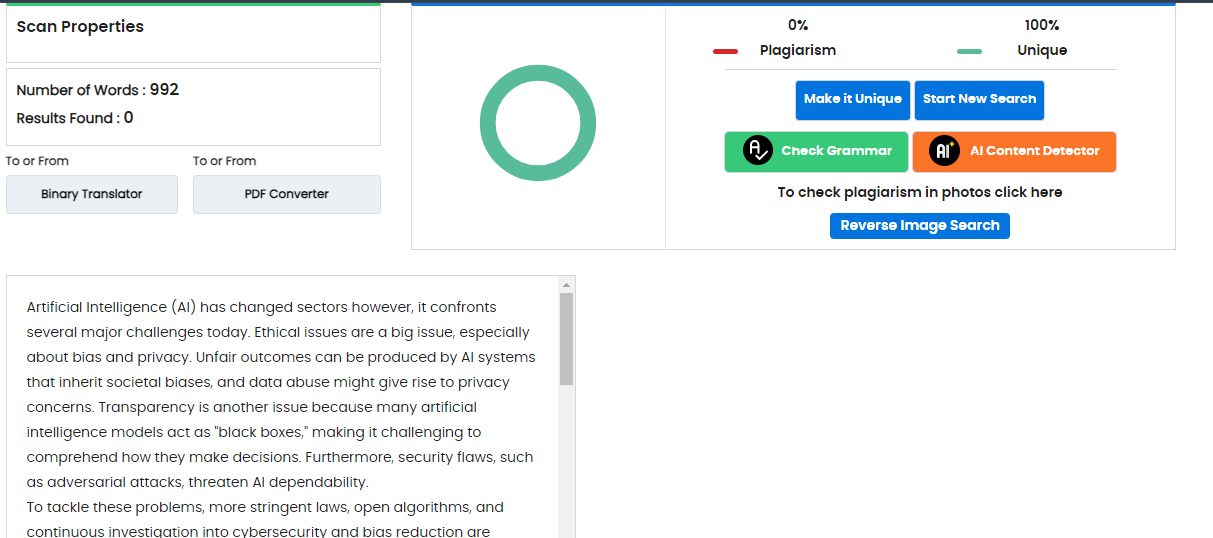